Introducing ClosedLoop - healthcare’s data science platform
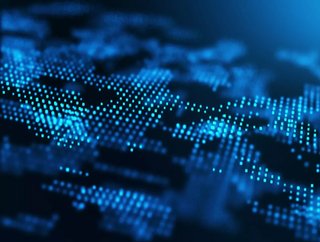
ClosedLoop is an Austin-based, AI-driven data science platform built for the healthcare sector. The startup recently beat competition from IBM, Accenture and Deloitte among others to win the Centers for Medicare & Medicaid Services (CMS) Artificial Intelligence (AI) Health Outcomes Challenge.
This $1.6 million award was given to the startup for its work in a national competition aimed at addressing the healthcare gap in the US. Carol McCall, Chief Analytics Officer, tells us what sets the company apart.
What does ClosedLoop offer?
ClosedLoop’s data science platform is purpose-built and dedicated to healthcare and life science organisations. The platform integrates four technology-enabled data science workflows: data onboarding and normalisation, automated feature engineering, model training and validation, and model deployment and MLOps. Each workflow embeds numerous capabilities and functions that facilitate exploration, collaboration, oversight, and management.
What makes ClosedLoop different to other AI platforms?
ClosedLoop.ai is healthcare’s data science platform. We make it easy for healthcare organisations (HCOs) to use AI to improve outcomes and reduce costs. Purpose-built and dedicated to healthcare, ClosedLoop combines an intuitive end-to-end machine learning platform with a comprehensive library of healthcare-specific features and model templates.
ClosedLoop’s customers use explainable AI to drive clinical excellence, operational efficiency, value-based contracts, and enhanced business results. What sets us apart is our commitment and ability to deliver unbiased accuracy and explainable AI solutions at-scale in healthcare.
How does ClosedLoop avoid bias?
The ClosedLoop platform has built-in capabilities for helping to address bias and fairness. This is crucial for AI systems, particularly when they are used to inform decisions about allocating limited resources. Models that systematically underpredict risk for a particular group can lead to that group being unfairly denied resources.
With respect to algorithmic bias, our AI platform systematically assesses for bias in model design, data, and sampling, and makes sure to use measures that are insensitive to differences in disease prevalence between groups.
To assess fairness, ClosedLoop developed a new metric called Group Benefit Equality (GBE). Standard fairness metrics are completely unsuited to healthcare situations - they ignore “false negative” errors, which can leave individuals who would benefit from an intervention unable to get it, or use arbitrary benchmark thresholds that fail to adjust for instances where the alarm rate for the reference group is too low.
The GBE metric addresses these shortcomings. GBE is also easily explained, has transparent procedures, and uses clearly defined thresholds to assess when models are biased.
What is explainable AI?
For ClosedLoop’s customers, Explainable AI is key because it completely reimagines the concept of patient risk profiling. It shifts away from legacy risk “scores” to a comprehensive, personalised forecast that can be delivered directly into a clinical workflow.
Each forecast - which might harness several years of patient-linkable data - surfaces key variables and explains precisely what risks a patient faces and why. Each forecast integrates relevant clinical information and can link to specific interventions that clinical teams use to prevent adverse events, improve outcomes, and reduce unnecessary costs.
How digitally mature does a healthcare provider need to be to use the platform?
The flexibility of ClosedLoop’s platform allows our customers to leverage AI-based solutions no matter where they are on the maturity curve of data science and machine learning. This is because our product has two main pillars of technology.
The first pillar is used by organisations with robust technical or data science teams, and includes a fully-featured automated data science and machine learning platform. The platform’s capabilities allows data science teams to move quickly, build bigger and better models, and operate at the top of their license.
It automates a lot of the mundane work and deep operational details so data science teams can focus on asking the right questions, engaging with clinicians and care management teams, and communicating results.
The second pillar is used by organisations without large (or perhaps any) data science or technical teams. They are able to quickly leverage the platform’s healthcare-specific comprehensive enterprise feature store and an extensive catalogue of off-the-shelf model templates for common healthcare use cases.
These allow customers to quickly train models with data from their specific population, fine tune them to fit the context in which they will be used, and deploy them rapidly into their operational workflows.
The company has won a number of awards, to what do you attribute its success?
One reason is because we have been bold. In the CMS Challenge, for example, we believed we had the right and the ability to win against some huge names. That becomes important when, along the way, advisors become sceptical and suggest we stay focused on the core business. Today, those same people have said, "Hey, this is big!" You have to make bets. You have to decide. While it’s true that the work was aligned with where we were going, the amount of effort it took was unreal. There's no other way to really take a shot than putting every hour of all your best people on it.
What goals do you have for the next few years?
ClosedLoop’s customers share a common goal of achieving the Triple Aim: improve patient outcomes, reduce unnecessary healthcare costs, and enhance the experience of care.
Achieving the Triple Aim has become a national imperative - nearly one-third of Medicare beneficiaries experience an unplanned hospital admission or other adverse event each year, and the US spends almost $1 trillion on healthcare annually that does nothing to improve health outcomes. ClosedLoop’s goal is to make it easy and affordable for every HCO in the country to become AI-enabled and to use our explainable AI solutions to achieve the Triple Aim.
- Elekta Uses AI to Support Healthcare in Rural CommunitiesTechnology & AI
- Converge International Leading way in Omnichannel TelehealthDigital Healthcare
- Nokia & STL Boost for Indian Healthcare NetworksTechnology & AI
- AI Tool 'Picks up Early-stage Breast Cancers Doctors Missed'Technology & AI