Key AI trends digitally disrupting healthcare
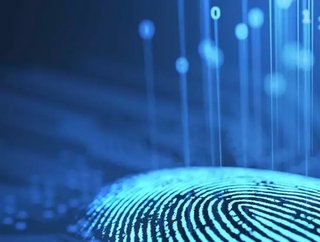
According to Global Head of Artificial Intelligence and Automation at Infosys, John Gikopolous, the healthcare industry is ripe for disruption by new and innovative technologies such as AI and machine learning. He explained to us that there are four key trends that could drive this change.
Intelligent Process Automation
“This is the central one,” says Gikopoulos. “Automation, RPA and IPA should really be running all of the various solutions in the background. From the payer perspective, it’s about collecting information, registering people, allowing one-point access to past information and so on; from the provider side, the entire experience in a hospital should be underpinned by automated processes. For pharma companies, for example, automation is used across all functions, from manufacturing and support, through IT, finance and even the running of clinical trials and R&D.”
The key advantage of RPA and IPA is the skipping of the human interface, according to Gikopoulos. Employing the technologies removes the delays or mistakes that humans make from the equation, thus making the entire value chain more efficient from end to end.
Standardising data
“The biggest problem across the entire healthcare value chain is being able to call the same thing the same name at every stage of the process, so underlying or diagnosed conditions of a patient, the effect of different treatments - or a lack of treatment - has had in the past and so on,” Gikopoulos explains.
“It drives every decision, from what active agents go into drugs and when to dispense then, through to clinical trials, how long people should be hospitalised and even what impact they may have on the greater public and the healthcare system.
“And yet, it’s a question that just hasn’t been addressed adequately,” he continues. “Information comes from so many sources in healthcare that standardising that information is essential. Further, once you have that standardisation, then you can apply AI to identify the questions behind that information.
Machine learning
Machine learning is used at the payer/patient or hospital/patient interface to analyse data, often provided by patients, and to provide informed reactions and decisions on that data.
“When you start looking at the outcomes of treating patients in different ways depending on when they came in - what symptoms they have, what were their underlying conditions, demographic or social category, then you define a completely different decision tree compared to the static, traditional one that says the customer journey or the patient journey is greet at the reception, triage, send to doctor, have it diagnosed and then send to be treated,” notes Gikopoulos.
He also cites the importance of machine learning in clinical trial efficiency, adding that it “could be grossly understated in certain cases”. These include, for example, identifying cause and effect in the use of different agents within drugs along the clinical trial value chain, which can work in a much faster and more targeted way with machine learning.
Patient interfacing
According to Gikopoulos patient interfacing can “completely change the experience that we all have from communicating with this entire value chain”. Examples he cites include using remote channels to contact patients that need a certain treatment after discharge, or the use of telematics to remote diagnose patients.
“Interfacing isn’t all about chatbots, avatars or cool looking bots that interact with you,” he says. “To a large extent, it’s more to do with the IoT and making us part of the connected world not just for the mundane and potentially also fun parts of our experience and existence, but also the more crucial areas like healthcare.
"Imagine, for example, if all our machines, computers, phones and so on, instead of using their enhanced abilities to provide greater gameplay, screens, or sound quality, had actually focused on having the type of sensors that allow temperature or blood pressure to be taken, or a person's retina to be scanned for specific diseases. That kind of interface or interaction could, or might still, completely change everything."
- Elekta Uses AI to Support Healthcare in Rural CommunitiesTechnology & AI
- Converge International Leading way in Omnichannel TelehealthDigital Healthcare
- Nokia & STL Boost for Indian Healthcare NetworksTechnology & AI
- AI Tool 'Picks up Early-stage Breast Cancers Doctors Missed'Technology & AI