Encord’s Andreas Heindl & Eric Landau on AI saving lives
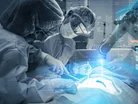
Over the past few years, the world has been stunned by what the healthcare industry has gone through. Healthcare professionals are quitting in high numbers and they aren’t all being replaced, adding more pressure onto those who remain. One thing that may be able to support healthcare workers during such times is the advent of AI.
“AI can help healthcare professionals by assisting with patient diagnostics, such as triaging, in which patients who require immediate medical attention are placed at the front of the line,” said Andreas Heindl, Machine Learning Product Manager at Encord.
Encord is a data-centric AI startup that offers users a platform to annotate, manage collaborative labelling and, ultimately, support control workflows.
Over COVID-19, many screening programmes were suspended. Doctors are now under a great deal of pressure to clear the backlog that has developed, which is one example of where AI can help.
“To ensure that the patient is in the proper position during the scan, an AI system can also be employed, which enhances the scan's quality,” Heindl continues. “It also lessens a patient’s stress if they are informed that a scan needs to be repeated. This saves the healthcare provider cost because the patient wouldn’t need to be recalled.”
Using AI to create democratised healthcare
Eric Landau studied Electrical Engineering at Stanford University and Applied Physics at Harvard University. Then, during his PHD, took a break to work in finance. It was during this time that he met Ulrik Hansen. They discussed the inefficiencies in data and, together, decided it was time to find a solution to empower the healthcare sector. They went on to found Encord in 2020, which is headquartered in London.
“My role at Encord involves solving a bunch of different problems and filling in gaps,” explains Landau. “As they say, I’m a ‘Jack of all trades, master of none’ – but that’s because my job evolves. There are new problems that crop up as you grow, so my job is to solve those new problems. It’s about always being bad at what you’re doing and finding an answer!”
Landau’s healthcare role model is the late Dr Paul Farmer, medical anthropologist and co-founder of Partners in Health.
“He studied at Harvard, then he worked in Haiti, helping community clinics to solve the TB crisis. Farmer’s work is the definition of democratising healthcare.”
Encord is built to support the machine learning (ML) community to do that.
“You want a computer to solve problems – to diagnose cancer or to detect patients falling from their beds. So we built a model that learns from data,” explains Landau. “Models are like children, they have no common sense, so we have to teach them.”
A model is, of course, only as good as its data, and Landau believes that this is how AI can save lives.
On a recent visit to one customer in Denmark, Landau got to see for himself how patient monitoring works in Danish nursing homes.
“There is a nursing shortage there,” Landau says. “The AI model detects and checks when there is an issue, to save the nurses time. Being preventative is a key element to the use of AI in healthcare, by solving problems before they proliferate. Another example is in automatic stroke detection and in improving Doctor-patient outcomes. A lot of Doctors’ time is spent making notes on paper; if you want your doctors to save time, AI can make those notes instead, indirectly saving lives.”
How AI is saving lives
To Andreas Heindl, Machine Learning Product Manager at Encord, AI isn’t viewed as something to replace the human, but to make them more powerful.
“Analysing lung scans takes hours for a human, but machine learning models can perform this task in minutes, streamlining and accelerating the process,” says Heindl. “The result is that doctors can focus their time on more complex tasks, such as developing patient care plans, and patients can gain access to health information more efficiently.”
If there isn't a domain expert available, Machine Learning (ML) can also be employed. In many isolated locations where individuals lack access to doctors, AI may be their only option to receive an accurate diagnosis.
“AI is great at acting as a second pair of eyes, and incidental findings are a common occurrence.”
Deep learning is significantly more data hungry than traditional machine learning. No longer do you create features, says Heindl, instead, you let the AI discover the best ones.
“Companies are realising that scaling up their annotation workflows is the issue because current state-of-the-art AI takes a large amount of training data. Data-centric AI is essential for reviewing, scheduling, and assessing the quality of both annotators and their output.”
In addition, harnessing automation can allow data labellers to become more efficient.
“Since a typical annotator has many years of experience in the industry, annotating data in the healthcare sector is expensive. As a result, AI businesses want to make sure that the annotation process is as straightforward as possible for them,” said Heindl. “It makes sense to automate repetitive tasks, like finding a tumour across multiple images.”
For instance, if the annotations for the first and final image of a tumour are provided, algorithms can automatically fill in the images in between.
“This accelerates the annotation process while also enhancing the platform experience for the annotator.”
Allowing healthcare professionals to do their jobs
Despite all this great innovation, problems persist.
“There are many problems,” Landau explains. “In getting your data to solve the problem, the biggest issue is the demographic bias.”
Demographic bias refers to the automated recognition of groups of people based on characteristics that are biological and behavioural.
“You might get data from one place, but it won’t work in another part of the country,” continues Landau. “Structured data sets that are balanced and unbiased will make them useful.”
Harnessing automation will allow data labellers to become more efficient. At Encord, that’s the goal for the healthcare sector.
“You get a lot more data out of this, more data and more time for the healthcare professionals to do the more important things – like looking after patients and their families.”
That’s what healthcare professionals and their patients want – and that AI can provide.