How AI can transform the future of genetic testing
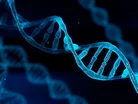
According to statistics, 300m people worldwide are affected by rare diseases, and about 80% of these are genetic in nature. Although rare in individual cases, these diseases collectively are not rare. Unfortunately, due to their complex clinical nature, they often go unnoticed and are poorly managed in the medical system. There’s great potential for Artificial Intelligence (AI) when it comes to analysing genetic data and supporting clinical professionals in making diagnoses. In this article, Dr Ansgar Lange, COO of Nostos Genomics, dives into developments and challenges for AI in genetic testing.
The challenge of assessing pathogenicity in rare genetic diseases
Advances in sequencing technology, such as the completion of the human genome sequence and the integration of Next Generation Sequencing (NGS) in clinical settings, have enabled clinicians to link specific genetic variants to rare diseases. Despite this, diagnosing rare genetic diseases still often ends in inconclusive results, as the pathogenicity of many genetic variants is still unknown (so-called VUS: variants of uncertain significance).
The paradoxical strength and weakness of modern genome-based sequencing technologies are that they provide vast amounts of data. This makes it challenging to identify one disease-causing variant from among millions of benign ones. Assessing the pathogenicity of a variant requires highly trained genomic specialists to comb through multiple lines of evidence, scientific literature, and disease databases. The rarity of individual diseases means that such evidence of a variant being pathogenic may never have been reported in a disease database. This can lead to discordance across laboratories and variant interpretation bottlenecks. This contributes to patients with rare genetic diseases often enduring years of health complications and emotional distress, waiting for a conclusive diagnosis and effective treatments. This is called the diagnostic odyssey.
Harnessing machine learning to accelerate genetic variant interpretation
To overcome these challenges, innovative machine learning algorithms have been developed that can comb through genetic datasets and support the identification of disease-causing variants within minutes. AI-driven tools are trained using molecular, experimental, population-level and clinical data sources interpreted by human genomic experts. The system can then learn this method of interpretation, predict candidate variants in undiagnosed genetic disorders and better inform healthcare professionals, supporting them in the labour-intensive task of variant interpretation.
By quickly identifying the most promising candidate variants for interpretation, AI-driven tools support experts in making fast, accurate and novel diagnoses to improve patient outcomes. These tools are simple to integrate and incredibly scalable, placing genetic laboratories in a unique position to scale their operations and keep up with the rapid growth of the market for genetic testing. This can be particularly beneficial for large-scale sequencing of populations, which produces large amounts of data that can overwhelm personnel resources in genomic laboratories. AI systems can help reduce the complexity and time of variant interpretation, providing an extra pair of hands and overcoming the interpretation bottleneck presented.
Overcome limitations of non-coding variants and ensure patient data protection
However, AI can only be as good as the data you feed it. Limitations arise in the form of non-coding pathogenic variants, ancestry-specific bias in genomic datasets and patient data protection issues. Non-coding variants are regions of the DNA sequence that do not directly code for proteins, making it more difficult to understand how mutations in these variants affect cellular function. To address this, AI is beginning to integrate experimentally derived data on non-coding DNA variants into their algorithmic training. This, in combination with variant data obtained from disease databases, provides a more extensive training platform for AI algorithms to learn and improve predictions.
The goal of these AI-driven tools is to support and enhance the expertise of clinicians, not to replace them. Regulatory and ethical issues can stem from the transparency of underlying algorithms themselves and the privacy of patient sequencing and clinical data. To ensure trust, governments or regulatory bodies will need to ensure clear rules for this and the proper implementation of AI-aware policies on how a patient’s genetic information is used.
A bright future for AI in genetic testing
AI is becoming more accurate and affordable, and with continuous advances in high-throughput sequencing, diagnosing a rare genetic disorder is becoming more economical and accessible for global healthcare markets to invest in. As the wealth of genomic datasets grows, access to this data will be vital in providing further training datasets for AI systems to excel. AI will diagnose better, faster and with less bias to fill the diagnostic gap for undiagnosed patients.
For clinical adoption of AI systems, it is essential that the trust of populations is won when it comes to participation in genetic testing and data sharing. Technology adopting white-box AI models can help, as these models have a core value of being understandable and interpretable, allowing for human-based explanations of predictions and increased understanding of how patient data is utilised. Furthermore, for AI to progress in a clinical setting, clear guidelines need to be defined for when AI-driven genetic sequencing is most appropriate for a patient.
As AI-driven interpretation correctly predicts disease-causing variants that previously had uncertain significance (VUS) in relation to disease, a revolutionary shift in how patients suffering from rare genetic disorders are diagnosed and treated is becoming increasingly possible. This shift will be bolstered by the successful implementation of increasing multiomics data, continued algorithmic transparency and progressive government guidelines. Through the successful integration of AI-driven tools, clinicians will be able to diagnose rare genetic diseases more efficiently and effectively, bringing hope to the millions of people affected by them worldwide.
Featured Articles
NTT and medical technology company Olympus put on a demonstration, showing the IOWN APN's low-latency capability, for the first cloud endoscopy system
Richter BioLogics GmbH & Co KG's a new biopharmaceutical GMP facility in Germany will boost production capabilities and local employment
A pilot study from GE Healthcare has shown the value of Portrait Mobile Continuous Monitoring Solution, a leap in post-surgical monitoring efficiency