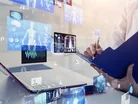
The benefits of artificial intelligence to healthcare are under scrutiny, with two researchers from Stanford University recently saying AI wouldn't have any significant impact until the 2030s.
“In the tech world, progress tends to happen slowly and then very quickly” Andrew Ng, founder of Google Brain and adjunct professor at Stanford said. However other experts dispute this. One study, carried out at a hospital in Wisconsin, found that using a clinical AI tool by Jvion reduced readmissions by 25%.
We asked Jvion’s Chief Medical Officer Dr John Frownfelter where AI can provide the most value, and how to overcome challenges such as algorithmic bias.
Is AI technology advanced enough to provide real benefits to healthcare providers right now?
It depends on the application. Some applications of AI, such as assisting with the interpretation of radiology images, still have a way to go before they have a transformational impact, mostly because the machine learning models need to be trained on the population they are used on. As soon as you bring the machine learning model to a different hospital, it needs to be retrained - a time consuming and resource intensive process.
Where is it currently delivering the most value?
When it comes to managing patient risk and providing clinical decision support, AI is already delivering significant value. As long as the AI model has access to a patients’ medical record and their address, it can map them to cohorts of similar patients along clinical, socioeconomic and behavioural lines, and based on these connections, predict which patients are on an avoidable path to an adverse outcome, such as an ER visit.
The AI can then present clinicians with a list of patients to prioritise for early interventions. By identifying the relevant clinical, socioeconomic, or behavioural risk factors for any given patient, the AI can also provide recommendations for evidence-based interventions that address these risk factors, enabling clinicians to take action.
Hospitals using this form of AI, including Jvion’s clinical AI, have seen adverse outcomes such as hospital readmissions and sepsis events decrease by 20% or more on average, and saved millions each year.
Which key areas of healthcare can AI be most useful to in the future, particularly in terms of post-pandemic recovery?
AI can be particularly valuable for determining which patients, who may have deferred necessary care during the pandemic, should be followed up with or brought in for an in-person appointment to mitigate their risk of an adverse outcome.
As the industry continues to shift towards value-based care and this new paradigm of “hospital at home”, AI will be necessary to “travel” with each patient across the continuum of their journey to identify health risks as well as the most appropriate care settings and preventative measures to mitigate complications.
AI that helps providers and payers proactively intervene with patients at risk will improve patient outcomes — ultimately saving costs and reducing performance-based fines that could slow down the industry’s financial recovery from the pandemic.
What barriers exist to its adoption?
AI has transformative power only when understood, communicated, implemented and adopted properly. From my experience, clinician skepticism is the biggest barrier to adoption. If nurses, care coordinators, doctors, or other users don’t trust the recommendations of AI or don’t see their value, then the benefits of AI implementation will not be realised. Change management planning and the leadership of clinical champions are essential to success, as is data that demonstrates the relative effectiveness of any newly implemented technology.
A poor integration of AI within clinicians’ existing workflows can be a source of frustration and a point of failure in any AI adoption. Nurses and other users should be included early on in any implementation process to make sure the interface is intuitive to use and meets their needs.
The perception that AI is a “black box” is another barrier to adoption. The process of data collecting and data analysis should be transparent to all stakeholders so they understand where the predictions are coming from and what factors are being analysed. This is essential to building trust.
Financial concerns can also be a barrier, although it should be noted that many hospitals have achieved millions in ROI after implementing AI, so in my view it is worth the investment.
How does the problem of AI bias arise, and how can it be prevented?
Bias in clinical AI is a valid concern and can be a potent barrier to adoption. Some forms of clinical AI have been found to favour white patients over black patients in the past, so this fear is not unwarranted. Bias arises when the data used to train AI is not representative of the patients it will ultimately be used on.
It can also be the result of existing biases in the data. For example, one prominent AI tool that was exposed for its bias was predicting future hospital utilisation based on patients’ historical health insurance claim data. Because black patients are historically underserved and receive less care than white patients, they have fewer insurance claims. So the algorithm was prioritising care for white patients over black patients with the same need for care.
Any possibility of bias should be actively considered, mitigated, and tested for throughout the development of clinical AI applications. Data used to train AI should be diverse and representative of the patients it will be used on.
It also should consider more than just a single, one-dimensional factor, such as insurance claims. By incorporating data on social determinants of health (SDOH), AI can consider external socioeconomic or environmental conditions that can present barriers to care or positive health outcomes, and provide recommendations on how to mitigate these external factors — the same factors that drive health disparities to begin with.