How Jvion's AI can lead to better end of life care
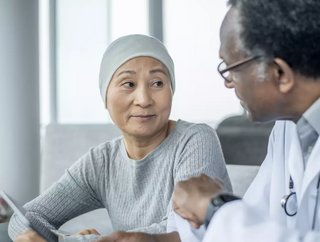
In a recent study, researchers found that an AI tool created by Jvion was able to predict patients with a high risk of dying in the next 30 days with more accuracy than those predicted to be at low-risk.
The Jvion CORE™ AI tool is already in use at practices in the US, including at Northwest Medical Specialties, where clinicians say it has helped double palliative care referrals, and led to more meaningful end-of-life conversations with patients who are terminally ill. Dr John Frownfelter, Chief Medical Officer at Jvion and co-author of the study tells us more.
How is the AI tool trained to predict mortality?
Our clinical AI repository, the Jvion CORE™, includes a vast database of over 37 million patient care journeys with around 4,500 data points on each individual. This includes clinical data but also data on social determinants of health (SDOH), such as whether a patient has access to transportation or lives alone.
From this database, our machine learning algorithms can group similar patients together, which we call similarity clustering. What this means is that we can match living patients with similar patients who ultimately died in order to predict mortality in the living. We then test the machine learning model by seeing if it can accurately predict the outcomes of a large, diverse, representative sample of patients whose outcomes are already known.
What factors contribute to the highest risk of mortality?
What we’ve found is that SDOH risk factors contribute more than expected. In particular, social detachment, limited transportation, and poor access to care resources are contributors to actual risk for mortality. Socially isolated patients are more likely to give up their will to live and stop taking their medication, for example.
The good news is that many SDOH risk factors are addressable, sometimes more easily than clinical risk factors. Because you can see patients holistically, the machine learning models recognise the combined effect of various SDOH pressures on a patient that lead to increased risk in combination with and in addition to obvious clinical risk factors.
How can this AI tool help drive earlier interventions, or towards palliative care services?
A patient’s risk often evolves faster than the oncologist’s understanding of their risk. So you end up with oncologists that are committed to a certain treatment path for a patient and haven’t necessarily considered when the patient’s trajectory demands a new approach.
Our AI identifies the risk that may not be obvious at a surface level, and gives oncologists a nudge that they may want to re-evaluate the patient and consider alternate treatment courses.
Is using AI in this way helping with patient choice?
Many patients do not get access to palliative care until it’s too late (68% are never referred for palliative care before they die). These patients die unexpectedly, without support from their care team, and without control of the process.
By predicting mortality well in advance, AI can prompt care teams to start conversations with patients about palliative care that they otherwise wouldn’t have.
To give an example, there was a breast cancer patient being treated at Northwest Medical Specialties (whose Medical Director Dr. Sibel Blau was also a co-author of the Future Oncology study) who was flagged as a high-risk for 30-day mortality by Jvion’s AI. The care team was surprised, she had been responding well to oral chemotherapy. But out of an abundance of caution, they had a friend drive the patient in for a blood draw anyway.
The patient seemed fine and was sent home. The care team called with the lab results a short time later and they learned from the friend that the patient had collapsed. It turned out she had a UTI that was progressing to sepsis. After a course of antibiotics, the patient recovered and was released home.
A few weeks later she came back in, and this time confessed that she was tired of chemo and didn’t want to continue. So she entered hospice care, and a few months later, died peacefully on her own terms.
What ethical questions surround using AI for end of life care decisions?
As with any AI use in healthcare, there is always an ethical concern around bias. However, the large and diverse sample size used to train our AI model (37 million patients care journeys) mitigates the inherent risk of bias.
Our approach is more true to real life, looking at patients holistically and including data on clinical factors but also behavioural, social, economic, and environmental factors that can have an influence on their health outcome.
The use of AI is in some ways more ethical than not using AI. It preserves patient autonomy, and can prompt physicians to pause and rethink what’s best for the patients. It’s also important to be clear that AI is augmenting a doctor’s decision-making — much like a lab test — not replacing it.
Because it’s a nudge, and not a directive, the AI avoids the unethical risk of AI inadvertently causing harm. It preserves the doctor-patient relationship, and in a way enhances it by prompting more honest and informed conversations that wouldn’t happen otherwise.
What do you predict the next innovations/greatest areas of impact will be for AI in healthcare?
From a tactical perspective, I think we’ll see more SDOH brought into the fold of AI. AI can be a powerful tool for bringing the thousands of potential SDOH risk drivers to clinicians’ attention in a digestible way. Otherwise, there is just too much data and not enough time with patients to get the full picture.
AI will also play a big role as healthcare becomes more virtualised. The pandemic accelerated a shift to telehealth and remote patient monitoring that is not slowing down. But between all the Apple Watches, bluetooth scales, smart beds and connected glucometers you need AI to assimilate all of the incoming data and present it to clinicians in a way that can drive their decision-making. Otherwise, virtual care will become impossibly complex to manage and more harm will result if all this data isn’t compiled and brought together.
Burnout is another area where AI can have a major impact. It’s a big problem that only gets worse as it drives skilled clinicians out of the workforce. A major driver of this burnout is the overwhelming amount of data clinicians have access to and the electronic demands of managing it all. AI can ease the cognitive burden on clinicians and enable them to spend less time in the EHR and more time with patients, which is why many went into healthcare in the first place.
Finally, AI will help better triage patients and focus resources on the patients at highest risk. For example, AI can identify those at lower risk, and automate touchpoints (like text messages or emails) via patient engagement platforms. This allows case managers to focus their time on higher risk patients for more in-depth phone calls, or to have those patients come in for in-person visits.
- Hologic: How Can Technology be Harnessed to Combat Cancer?Technology & AI
- Elekta Uses AI to Support Healthcare in Rural CommunitiesTechnology & AI
- Converge International Leading way in Omnichannel TelehealthDigital Healthcare
- Nokia & STL Boost for Indian Healthcare NetworksTechnology & AI