How Kintsugi uses AI to assess speech and diagnose anxiety
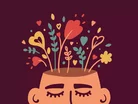
Kintsugi is an AI-based mental health platform that helps doctors detect depression and anxiety in patients by analysing their speech. We asked Kintsugi's Chief Medical Officer Prentice Tom how it works.
How can depression and anxiety be detected in someone's voice?
Producing speech involves complex coordination of various cognitive and motor processes, therefore, samples of speech provide insight into the state of patients’ physical and mental health.
Speech has long been studied for a variety of health conditions including dementia, Parkinson’s, Alzheimer’s, and psychiatric conditions. It has also been studied in clinical and academic works as early as 1921, and only in the last decade have advancements in deep learning provided a new lens in which we can further these long-studied works.
The way someone speaks - rather than what they say - provides a way for practitioners to listen with a “psychiatrists’ ear” for depression and anxiety markers.
How is the algorithm trained to detect this, and what rate of accuracy does it have?
Our algorithm (KiVA) is trained on the world’s largest annotated database for machine learning and mental health from clinical, academic, and commercial collaborations. Currently, we present 70 to 80% accuracy in detecting clinical depression and anxiety depending on the quality of the audio sampling and expect gradual improvements as we continue to draw from diverse populations and call environments worldwide.
Is it intended to be used in addition to other methods of diagnosis?
Both. The algorithm can be used as a standalone screening tool, however, like most instances in medicine, we rarely rely on a single test to define a patient's conditions. For example, when diagnosing diabetes, a doctor would factor in results from blood sugar tests, renal function, and small vessel disease, not just one definitive data set. It's the same when diagnosing anxiety and depression.
Kintsugi's algorithm should ideally be used with other diagnostic modalities and surveys, and possibly even other science that hasn't yet been developed to get the most robust clinical picture.
What benefits does Kintsugi bring to patients, and to clinicians?
There are three areas that Kintsugi benefits most: highlight underdiagnosed cases of depression and anxiety, making the health system aware of resource needs, and forming a more patient-centric model of care.
Currently, only 4% of primary care patients are screened for depression or anxiety - making mental health disorders one of the most underdiagnosed in medicine. The problem is that clinicians don't have physical findings to recognise the conditions: studies aren't always accurate, and clinicians can disagree on diagnoses without quantifiable metrics to confirm the decision.
At the same time, 80% of people with a chronic disease suffer from depression. As the population ages and elderly demographics grow, it's a safe assumption that there will be higher rates of depression and anxiety. Likewise, anxiety is becoming an epidemic among younger generations.
With only 20 seconds of free-form speech, Kintsugi tech provides a non-invasive resource for the elderly and youth to receive a diagnosis, improved care, and reduced suffering.
Our tech also significantly reduces the cost of care. 65% of recurrent emergency department users suffer from mental health disorders, as mild exacerbations of symptoms tend to mean people seek care when it's not an emergency. If these individuals can be identified and treated earlier, they wouldn't need to access healthcare as frequently, and thus the cost of care would be much lower.
What other areas of mental health can AI benefit?
The potential for AI in mental health is huge - it can outperform humans in a variety of ways, plus boost scalability, access, and equitability in existing tools, as well as lower costs and improve labour force efficiency in medicine as a whole.
AI already supports clinician decision-making, allowing us to use a broader, less expensive labour force to evaluate and care for patients. We can automate best practices, meaning we don't need the same volume of clinicians, nor the same level of training for clinicians. As such, we can tier workforces based on services that require different skills, experience, and knowledge, ensuring that patients get the right care according to their circumstances.
On top of that, AI helps create uniformity and quality in care. Studies show that clinician bias is real, whether intentional or not, and that AI-supported care can contribute to eliminating those biases and generate fairer, reproducible care. Admittedly, all AI needs further quality assurance to combat bias in its own algorithms, however, this bias can (and will) be corrected, while human bias cannot be corrected and automated in the same way.