The true diagnostic potential of AI
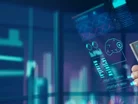
Ask tech wizards and healthcare industry leaders which innovations will shape the future of medicine for decades to come, and you’ll invariably hear about the revolutionary potential of artificial intelligence (AI).
We’re not far off, the story goes, from a world where algorithms play an integral role in augmenting the work of clinical professionals by evaluating medical scans with near-perfect accuracy, predicting prognoses, and helping identify personalized courses of treatment that will dramatically improve patient outcomes.
Among the most recent data points fuelling optimism about the future of AI-driven medicine was a major 2020 study by researchers at University College London and Babylon Health. The researchers found that a so-called counterfactual algorithm – one that predicts outcomes after testing whether changing certain variables would yield the same result – outperformed 75% of doctors in accurately diagnosing patients.
But while the study offered yet another demonstration of AI’s capabilities in clinical settings, whether patients and providers will be able to experience the benefits of this innovation at scale depends on how well these algorithms are constructed and how easily they can be deployed.
AI in healthcare: we’ve come a long way
That so much of the conversation now centres on AI deployment attests to how impressively AI has already performed in numerous studies. The question isn’t whether AI adds immense value to medicine. It’s how the healthcare industry can scale AI algorithms so that healthcare solution providers, hospitals, and medical facilities can deploy AI without busting their budgets.
If the industry can overcome the scalability challenge, it stands to reap major benefits. Across use cases, algorithms have shown remarkable power to predict diagnoses efficiently and accurately.
In 2017, a machine learning algorithm accurately predicted 7.6% more cardiac events than the approved method of the American College of Cardiology and the American Heart Association, while generating 1.6% fewer false alarms.
Two years later, a team of researchers at Google and several academic institutions announced that AI had detected lung cancer with a 94% accuracy rate, outperforming six radiologists and yielding fewer false positives or negatives.
Meanwhile, researchers at Imperial College of London study found that AI could diagnose the severity of small vessel disease in elderly patients with 85% accuracy, providing clinicians with vital insights in their efforts to detect and treat this degenerative brain condition more effectively.
Such advances – and more are coming – underscore just how well-crafted and sophisticated today’s deep learning algorithms are. But the enormous computing power required to run them poses a formidable obstacle to widespread deployment, raising the question of when promising clinical results will translate to real-world solutions for patients and clinicians.
The deployment challenge
Simply put, legacy hospital devices are ill-suited for AI deployment. Much of the hardware that legacy systems run on can’t handle deep learning algorithms’ intensive computing power requirements – a problem that will only get worse as algorithms get larger and more complex (i.e., even more accurate and sophisticated).
Even if hospitals deploy algorithms, scaling them across other devices remains a big hurdle. Take IBM’s recently developed AI model that interprets X-rays at resident radiologist levels. If the power required to run the model proves excessive and can’t be deployed across all devices, then all we’re left with is some very advanced technology that’s out of reach for all but the wealthiest healthcare systems.
Further complicating scalable deployment is that AI processing often occurs on on-site servers; while computing tasks can be offloaded to the cloud, cost and general privacy concerns make the cloud a less than ideal solution for many healthcare facilities.
Reaching scalability
Steep as the challenge is, AI can fulfill its diagnostic potential with a new approach to deployment – which can only be achieved through better algorithm design. Well-constructed algorithms that consider not only accuracy, but deployment from the get-go will benefit the entire medical supply chain, enabling commercially viable, scalable solutions for any environment. Improved algorithms should be the path we take, as opposed to requiring hospitals to buy updated hardware every time they need new algorithms deployed.
Developers must ensure that the algorithms they create can be easily deployed, whether it be in the cloud or on a hospital’s device itself. Consider GE Healthcare’s innovative algorithm for assessing Endotracheal Tube (ETT) placements in COVID-19 patients. The algorithm itself is worthy of awe – but what’s even more significant is that it has been added to GE’s Critical Care Suite 2.0, a collection of AI algorithms for easy deployment on mobile X-ray devices.
For all the promise AI is already showing as a diagnostic tool, it’s still in its very early days. Grand View Research forecasts that the global AI diagnostics market will reach $3 billion by 2027, up from $288.1 million in 2019 – an increase of nearly 950%. Better algorithms built with deployment in mind will play an indispensable role in that exponential growth over the coming years.