How poor data governance holds healthcare organisations back
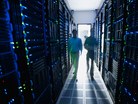
The widespread adoption of the value-based care model is encouraging more healthcare organisations to revisit the management of their data. Increased emphasis on the quality of service, elevating care outcomes along the way, means that organisations depend more than ever on consistent, accessible, and high-quality data.
The problem is that the current state of data management is inconsistent and disorganised. Less than half of healthcare CIOs trust the current quality of their clinical, operational, and financial data. In turn, the low credibility of their data sources calls into question their reporting and analytics, which ripples outward, inhibiting the entirety of their decision-making. Clinical diagnoses, operational assessments, insurance policy designs, and patient/member satisfaction reports all suffer with poor data governance.
Fortunately, most healthcare organisations can take straightforward steps to improve their data governance – if they are aware of what’s hindering their reporting and analytics. With that goal in mind, here are some of the most common challenges and oversights for data governance and what your organisation can do to overcome them.
Data silos in healthcare
Most healthcare organisations are now aware of the idea of data silos. As a whole, the industry has made commendable progress breaking down these barriers and unifying large swaths of raw data into centralised repositories. Yet the ongoing addition of new data sources can lead to the return of analytical blind spots if your organisation doesn’t create permanent protocols to prevent them.
Consider this situation: Your billing department just implemented a live chat feature on your website or app, providing automated answers to a variety of patient or member questions. If there is not an established protocol automatically integrating data from these interactions into your unified view, then you’ll miss valuable pieces of each patient or member’s overall story. The lack of data might result in missed opportunities for outreach campaigns or even expanded services.
Adding any new technology (e.g., live chat, healthcare diagnostic devices, virtual assistants) creates a potential threat to the comprehensiveness of your insights. Yet by creating a data pipeline and a data-centric culture, you can prevent data siloing from reasserting itself. Remember that your data ecosystem is dynamic, and your data governance practices should be too.
Lack of uniformity in healthcare data
None of the data within a healthcare organisation exists in a vacuum. Even if the data within your EHR or medical practice management (MPM) software is held to the highest quality standards, a lack of consistency between these or other platforms can diminish the overall accuracy of analytics. Worst of all, this absence of standardisation can impact your organisation in a number of ways.
When most people think of inconsistencies, it probably relates to the accuracy of the data itself. There are the obviously harmful clinical inconsistencies (e.g., a pathology report indicates cancerous cells are acute while a clinical report labels them chronic) and less glaring but damaging organisational inconsistencies (e.g., two or more different contact numbers that hamper communication). In these examples and others, data inaccuracies muddy the waters and impair the credibility of your analytics. The other issue is more subtle, sneaking under the radar: mismatched vocabulary, terminology, or representations.
Here’s an example. Let’s say a healthcare provider is trying to analyse data from two different sources, their MPM and their EHR. Both deal with patient demographics, but might have different definitions of what constitutes their demographics. Their age brackets might vary (one might set a limit at ages 18 to 29 and another might draw the line at 18 to 35), which can prevent seamless integration for demographic analysis. Though less harmful, this lack of uniformity can curtail the ability of departments to have a common understanding and derive meaningful business intelligence from their shared data.
In all of the above instances, establishing a single source of truth with standardised information and terminology is essential if you’re going to extract accurate and meaningful insights during your analyses.
To combat these problems, your organisation needs to decide upon a standardised representation of core data entities that create challenges upon analysis. Then, rather than cleansing the data in their respective source systems, you can use an ELT process to extract and load structured and unstructured data into a centralised repository. Once the data has been centralised, you can evaluate the data for inaccuracies, standardise the data by applying data governance rules against it, and finally normalise the data so your organisation can analyse it with greater uniformity.
Poor accessibility for data governance in healthcare organisations
Even when your data is high-quality and consistent, your organisation might still fall short of data governance best practices. The reason why? The accessibility of your data might not thread the needle between HIPAA compliance and appropriate end-user authorisation.
Some organisations, dedicated to protecting the protected health information (PHI) of their patients or members, clip their own wings when the time comes to analyse data. In an attempt to avoid expensive HIPAA violations, they restrict stakeholders, analysts, or other covered entities from accessing the data. Though it’s essential to remain HIPAA compliant, data analysis can be conducted in ways that safeguard PHI while also improving treatment quality or reducing the cost of care.
Your organisation can de-identify records (removing names, geographic indicators, contact info, social security numbers, etc.) in a specific data warehouse. Presenting scrubbed files to authorised users can help them gain a wide range of insights that can transform care outcomes, reduce patient outmigration, reduce waste, and more.
Elevating your overall data governance in healthcare
With all of these challenges in sight, it’s easy to get overwhelmed about the next steps. Though we’ve provided some actions your organisation can utilise, it’s important to recognise effective data governance is as much a change in your mindset as it is a series of best practices. Here are some additional considerations to keep in mind as you work to improve your data governance:
You need a defined data governance strategy
An ad hoc approach to data governance will fail in the long run. There needs to be agreement among your data stakeholders about data availability, consistency, and quality. Often, it helps to start with a pilot project on a single line of business or department to ensure that all of the kinks of the transition are ironed out before your data governance strategy is taken enterprise wide.
Even then, compromise between standardisation and distributed action is important so users within your organisation are following the same best practices as they conduct dispersed analytics.
Your healthcare organisation culture likely needs to change
Eliminating data inconsistencies or adjusting inaccuracies are temporary fixes if only your executives are committed to making a change. Employees across your organisation need to embrace the ideals of effective data governance if your organisation is going to gain useful and accurate intelligence from your data.