Clinical trial design: When age is more than just a number
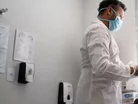
Dr Gen Li, CEO and Founder of Phesi, discusses the impact of Covid-19 on clinical trials - and the significance of age
Perception-led rather than data-led clinical trial design still lies at the core of many challenges in clinical development. Moreover, the challenge of clinical trial design does not discriminate between the smallest biotech startups and the largest pharmaceutical companies. All organisations in the clinical development sector suffer from similar challenges. We can confidently say that there is no perfectly designed clinical trial. But even for those trials that complete successfully, there are still design elements that could have been improved – reducing patient burden and operational cost, and accelerating time to commercialization or a “stop” decision. And the breaking news? Many if not most of these outcomes could have been predicted.
COVID-19 or design issues?
Numerous existing problems in trial design have been exacerbated by the COVID-19 pandemic, but not caused by it.
Throughout the past 18 months, we have seen several examples of trials thought to be at risk of failure because of the impact of the pandemic. On closer review, the reality is that these trials were already at risk because of factors rooted in design issues. When a trial ran into problems and needed rescue, it was typically because investigator sites were not recruiting patients at the speed they should be.
To solve this problem, the easiest solution appears to be adding more investigator sites and hoping that those sites recruit more successfully. However, simply adding more sites does not solve the problem, in fact it adds unnecessarily high costs to a study and compounds the trial performance issue. More often than not, investigator site enrollment performance is the symptom, while the root cause is the trial design itself. Successful trial design relies on identifying variables and trends in the patient population and using structured and integrated global data to do this. One such variable is patient age distribution.
Tracking dynamic age patterns
There is a wealth of historical trial and patient data available that can be employed in study design. In analysing 4,000+ human diseases, it becomes clear that each disease has a distinct pattern in patient age distribution. This pattern is dynamic. It is also predictable. It can be positively impacted by medical innovations and varies geographically as socio-economic factors differ. Age should always be an integrated part of trial design. However, many organisations lack access to sufficient volume of relevant data, and so create patient age eligibility parameters which are inaccurate.
This typically results in numerous protocol amendments being made while implementing the trial, with concomitant and significant financial costs, and prolonged cycle times – and even the potential failure of a trial. Recent analysis by Phesi of Phase III trials showed that 9% of undergo protocol amendments to modify patient age eligibility. This figure is 8.9% for Phase II trials. Given that Phase III trials are defined as “confirmatory”, this similarity is alarming. Applying insights from data can optimise clinical trial design relating to patient age eligibility and potentially avoid such protocol amendments. The following example using protocols for a neurological disease clinical trial demonstrates how.
Case study – neurological disease clinical trials (source: Phesi analysis):
Synthesised patient characteristics from 69,495 patients with this neurological disease show the average age of patients was 60.9 years old, with standard deviation of 9.5 years (fig.1.). Among these patients, 31% were over 65 years old. The average disease duration was seven years. There were very few patients under 40, ranging between 0.4% and 3.5%. For the combined cohorts, 1.5% patients were under 40 years old (37 out of 2,384) (fig.3.).
Unsurprisingly, most of the clinical trials for this neurological disease included patients 40 years and older (105/198) (fig.3.). Indeed, 40 years old is the modal age value (i.e., the data value that appears most often in historical trial data and so helps to identify potential anomalies that may cause failure or delay).
However, despite the modal value, 16% of protocols included patients 18 years and older (32/198). This is a design element risk. Typically, where design elements are outside of modal values, we subsequently see protocol amendments implemented to bring the ranges back in line. This proved to be the case in this example, with multiple amendments for age eligibility made (fig.4.).
From analysing synthesised patient profiles in this neurological disease, it becomes immediately clear there are few patients between ages 18 years and 40 years. Furthermore, reviewing historical protocol amendments data shows us that protocols are eventually amended to conform with the modal value of 40. Had the trial sponsors in these 198 protocols accessed this data, many protocol amendments could have been avoided.
Data-driven trial design
Making use of modal values requires significantly large pools of historical and current trial data to find the most commonly used protocol elements including:
- Age
- Gender
- Treatment duration and other comparators
These data points are then used to apply modal value-based design. Such a data-driven approach will reduce the number of amendments; reduce the cost of trials and reduce cycle times. Ultimately, it will accelerate the path of a treatment from development to market, assuming safety and efficacy milestones are met.
This requires a blend of data science and medical expertise. It also necessitates investment in digital analytics, with a combination of:
- Machine learning
- AI
- Natural language processing
- Integrated algorithms
Although it may seem complex to implement, modal value-based trial design has the potential to revolutionise the ways in which we plan and implement clinical studies.
What’s more, today, the industry has access to large volumes of published data, publicly available data, and historical trial data that can now be analysed via predictive analytics to optimise trial design and make modal value guided design possible.
With such data at their fingertips, now is the time for sponsors to focus on how to employ it to their advantage – and ultimately accelerate getting critical therapies to patients.
Featured Articles
NTT and medical technology company Olympus put on a demonstration, showing the IOWN APN's low-latency capability, for the first cloud endoscopy system
Richter BioLogics GmbH & Co KG's a new biopharmaceutical GMP facility in Germany will boost production capabilities and local employment
A pilot study from GE Healthcare has shown the value of Portrait Mobile Continuous Monitoring Solution, a leap in post-surgical monitoring efficiency