Utilising AI to improve rare disease drug development
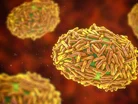
The development of treatments for rare diseases has always been subject to significant challenges, with limited patient populations, lack of research and unknown disease progression and a lack of investment to name a few. On average, it takes over four years to receive an accurate diagnosis of a rare disease and, despite their prevalence being scarce individually, currently, 1 in 17 people in the UK are affected by one of over 7000 rare diseases in the UK.
Developing treatments for rare diseases can be expensive with limited financial incentives making them less attractive for pharmaceutical companies to invest in when compared to more prevalent diseases. Additionally, due to the limited patient population for investigation and potential safety concerns, regulatory agencies may require more rigorous data to support approval of treatments in rare diseases. Although incentives exist for developers in the rare disease space, the UK’s current drug discovery process can be slow and prone to high failure rates and comes at a high cost to pharmaceutical companies.
Considering the hurdles facing development of effective treatments for rare diseases, the recent government announcement to invest almost £1m on projects to increase investment into rare disease drug development through the England Rare Disease Action Plan has been welcomed, however there was no mention of implementation of technological advances, such as AI.
AI and Machine Learning (ML) offer several opportunities in rare disease drug development in the UK, and must become a primary area of focus to ensure an effective and innovative way to improve the chances of finding effective treatments for rare diseases.
Leveraging AI for early detection
Early detection is key to ensuring the likelihood of a good prognosis for patients living with rare diseases. However, unfortunately, this isn’t common, with the average timeline of diagnosis of rare disorders being between 4-5 years, and even a decade in some cases, meaning patients have long waits for what could be lifesaving treatments.
AI has the potential to revolutionise the way rare diseases are detected and can be used to identify patients with rare diseases by analysing electronic health records, medical images and genetic data. Recent studies have found AI to be 90% accurate in diagnosing rare diseases in infants.
While further research is, of course needed, the fact remains that early detection of these conditions can enable more precise diagnosis and more timely treatment, leading to improved patient outcomes.
Lucy Radley, Senior Vice President, Head of European Regulatory Development at ProPharma Group
Automating clinical trials
Conducting clinical trials for rare diseases is incredibly complex and getting it right from the beginning is critical for both the stakeholders involved in the trials and the patients. Machine learning and AI have the potential to transform clinical trials by designing and automating aspects of the process by identifying the most suitable patients, predicting outcomes and optimising dosages and regimens of drugs.
AI algorithms can also be used to automate the collection of patient data, such as medical records and laboratory tests, and to analyse this data in order to identify potential treatments. For instance, data analysis is an area of clinical trials that can hugely benefit from automation. ML algorithms can provide many insights into the data collected from clinical trials, both during and after testing. This allows prediction of patient behaviour and adverse events from the data extracted by the algorithm. Similarly, the dataset conversion process, which is usually quite time-consuming and expensive, can be automated to bypass the need for complex programming and increase the likelihood of meeting regulatory requirements.
AI technology that is specifically designed to automate the process can not only reduce the cost and time associated with drug development, but can also optimise the design of the trials themselves, helping to reduce the number of patients required and increasing the accuracy of the results.
Improving drug development
Currently, the healthcare industry has had limited success when it comes to drug development for rare diseases and shockingly, only 6.2% of orphan drug developments reach the market. Many organisations prioritise large patient populations in traditional drug discovery processes as they enable a deeper understanding of disease biology. This is primarily due to the fact that there are simply fewer patients to provide the insights needed to develop treatments for rare diseases.
When it comes to the testing and synthesising of new drugs, AI can streamline the process for optimal efficiency, by creating virtual models of new compounds for more accurate testing and by repurposing existing treatments for rare diseases. By leveraging AI to improve drug development, it is possible to reduce the cost and time associated with drug discovery and development.
In conclusion, AI technologies continue to transform industries, and the opportunities for innovation within the development of treatments for rare diseases and the wider pharmaceutical industry are endless. It is vital that governments and funding bodies continue to invest into the technology to aid us to detect these complex diseases as soon as possible and to improve the efficiency of treatment. Equally important is the evolution of the regulatory framework alongside the use of AI and other such innovations in drug development. Our ability to innovate and use new technologies to our advantage can lead to a better outcome for all if we can revolutionise the drug discovery process.