Natural Language Processing unlocks hidden data to transform healthcare efficiency, quality and cost
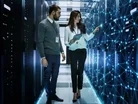
The digitisation of health care has created an explosion of data. Growing patient volumes due to an aging population and greater access to health insurance have combined with increasing adoption of electronic health records (EHR) and IoT, remote monitoring and wearable devices to spawn a massive influx of information.
This sea of data has tremendous potential to improve the quality, efficiency, and cost of healthcare. For providers, it could mean greater detail in documentation, more efficient diagnoses, and access to broader, potentially more effective, treatment recommendations. For payers, this data could provide insights that improve decisions about patient care within the confines of coverage limitations and help inform adjustments in health plan product offerings. And, for patients, it could mean more efficient access to care and more effective treatments at a lower cost.
The Data Dilemma
But, making sense of it all is a formidable challenge, and right now, payers and providers are often forced to make important decisions without access to the best possible data. The hurdle: as much as 80% of meaningful clinical data is captured in unstructured data, including physician notes, clinical observations, family and social history, etc.
Within this data lies powerful insights that both physicians and payers could use to make better decisions. The problem is that analysing this unstructured data typically requires manual, human review, which can be error-prone and resource-intensive. This productivity problem leads to immeasurable waste and operational inefficiency, missed revenue, higher costs, longer time to diagnosis and treatment, and overall diminished ability to manage patient and population health.
NLP: Solving the Productivity Problem
Natural language processing (NLP) has emerged as a promising solution to the problem, creating an efficient, effective way to understand and analyse this unstructured data. By unraveling the complexities of human language using artificial intelligence, NLP solutions can interpret the expressiveness, variety, ambiguity, and implied meaning of physician dictation, notes and other clinical unstructured data to make this data usable in making care and payment decisions at massive scale.
For health care applications, NLP’s value lies in its ability to understand grammar, syntax, context and intent, specifically around the esoteric nature of clinical language. It must understand complex medical jargon, organisation-specific language, the abbreviations and shorthand, etc., found uniquely in health care environments. For example: does the abbreviation “AF” represent the patient’s initials, atrial fibrillation, or amniotic fluid?
A clinically-oriented NLP can decipher coding based on the context within the patient’s chart, language parsing, named entity recognition, part-of-speech tagging, sentiment analysis, and disambiguation. An effective health care-specific NLP can record and document problems and negated problems with contextual attributes and even assign a degree of certainty and temporality, all automatically and in a format that can be usable for multiple applications. Here are just a few broad examples:
- Population Health
NLP analysed data can help physicians make faster, better-informed and more accurate clinical decisions. By having access to unstructured data from multiple sources, such as lab results, office visits, and diagnostic notes across the care continuum, physicians can better identify at-risk patients, spot chronic conditions, and devise better treatment strategies based on a more comprehensive and clearer picture of the patient’s overall health and wellness. At the same time, this data can ensure more accurate coding and billing, and aid in outcomes-based/bundled payments programs, as well as regulatory reporting for quality assurance.
See also
- From finance to healthcare: 5 fintech trends that will benefit digital health products
- AI is seen less of a threat and is welcomed by health professionals, research reveals
- Leveraging technology and education in the high-stakes fight against fake medicines
- Research
By leveraging population data analysed through NLP, research entities can gain a broader perspective when it comes to drug development and clinical trials. For example, NLP can be used to conduct pharmacovigilance for adverse event monitoring by culling through clinical reports of patients with an efficiency and speed no human analyst could match. It can also be used to assemble trial cohorts by identifying patients who meet specific criteria for participation, but who also don’t possess any disqualifying factors. In the trial process itself, NLP can make analysing all data collected exponentially faster, all of which can help to make safer, more effective drugs and other treatment therapies available much faster.
- Coding & Documentation
NLP’s supercharged efficiency really shines when it comes to speeding documentation processes. Not only can it facilitate computer-assisted physician documentation and automate the creation of patient summaries, but it can also enable computer-assisted risk adjustment coding. This not only speeds the documentation process and ensures that all diagnoses and procedures are coded, but also enables more efficient billing and payments.
In the case of government-sponsored health programs, such as Medicare Advantage or Affordable Care Act plans, risk adjustment is a tedious, manual process by which coding specialists review records for unreported risk conditions. NLP can streamline this process by automatically reviewing every chart, alongside other EHR data and surfacing those where errors may be present. This enables systematic retrospective review of only those records where it’s truly necessary to eliminate waste, increase coder productivity and optimise ROI on the risk adjustment opportunity. At one large, provider-sponsored health plan, this process cut the number of member reviews by nearly 80 percent, generating a 4X increase in coder capacity and over $650,000 in incremental revenue.
On the flip side, NLP can also mitigate compliance risk by identifying previously claimed codes without substantiating evidence in medical records. This helps provider and payer organisations to improve accuracy and reduce waste. In one health plan case, an NLP review of records helped to identify and correct over 120 non-compliant codes.
The Reality of AI
Of course, no computer-assisted, automated or AI solution is without some risk or drawback. Now that we’ve covered some of what NLP can do, let’s be clear about a few challenges that remain.
- Lack of EHR integration. In most cases, NLP is still a step removed from EHR and has yet to be integrated into the physician’s workflow on a large scale. Achieving that integration would dramatically accelerate manual tasks and give physicians more clinical time for direct, hands-on patient care. Current methods have physicians spending far too much time on charting and documentation, whereas integrating NLP technology could reduce much of that burden, allowing physicians to treat more patients and engage in more meaningful interactions.
- Overcoming data silos. Having access to complete data for each patient as they move through various parts of the care continuum is still a challenge. Without connected systems linking a primary care physician, specialists, hospitals, labs and more, any NLP will be limited in its capability to deliver the breadth and depth of data that can transform the health care experience. While NLP can still operate within data silos, eliminating them by connecting data can unlock even more value.
- Processing quality. All NLP technologies are not created equally. There are a number of NLP technologies in place in many other industries that simply don’t translate well to a health care application, and most health-specific NLP engines aren’t built to adequately handle the rigors of healthcare. Quality of data processing is critical, and analysis must be accurate. Not only are we dealing in potentially in life-and-death decisions, but inaccurate results do more to impede the efficiency of care by forcing providers and payers to double-check everything. Health care NLP engines must be able to provide reliable performance at scale, not only in terms of processing power, but also accuracy. A well-trained, fully calibrated NLP can deliver dependable results.
A Promising Future
While NLP and other AI solutions for health care will never eliminate the need for clinician review, expertise and validation, they can certainly accelerate the process by providing a concise, yet thorough, summary of evidence that can be used to make faster, smarter treatment and payment decisions. Implementing NLP solutions can enable health care organisations to greatly improve efficiency, accuracy and access to care, thereby improving patient outcomes and overall population health.
Credit: Anand Shroff, Chief Development Officer at Health Fidelity
- Elekta Uses AI to Support Healthcare in Rural CommunitiesTechnology & AI
- 48% of women say work has a negative impact on mental healthDigital Healthcare
- Digital healthcare with OSF HealthCare & Current HealthDigital Healthcare
- Birdie’s digital home care supports older adultsDigital Healthcare